Getting data from one place to another can be a time-consuming task and leads to revenue losses. With the help of Optical Character Recognition (OCR), businesses can automate this process, reduce errors, and mitigate the possibility of incomplete data as this technology can convert handwritten text and printed images containing text into digital and machine-readable formats.
Challenges
- Data volume and diversity – The amount of data produced, along with the volume of documents to be processed, has multiplied exponentially over the past years and will continue to increase in the future. With the explosion of unstructured data and large volumes of documents with such data in various forms and formats for processing, a traditional OCR cannot cope with this diversity as it is based on rules and templates.
- Cumbersive setup process – The OCR setup process can be lengthy and expensive and each alteration requires new rules and templates. It also requires manual efforts for template settings.
- Lack of flexibility and automation – Lack of flexibility in processing documents like invoices, which have high variability, leads to low accuracy levels due to the error streams. Additionally, not being able to fully automate the process of capturing and processing documents might lead to continuous alterations in the rules and templates

Solutions
A recommendation engine is built on a variety of factors to generate the best possible value for its users. Most of these include collaboratMachine learning and deep learning are promising technologies for extracting and analyzing large amounts of unstructured data. These technologies can analyze the data to learn and perform various tasks or processes automatically based on the findings. AI is a game-changer for OCR in the following areas:ive filtering and content-based analysis.

Document classification – Machine learning and deep learning power up an OCR system to automatically classify (and distinguish) documents based on their contextual information like form and format (PDF, jpg, png, etc), structure (unstructured, structured, or semi-structured data), and type (check, invoice, label, bank statement, etc).
Data extraction – Apart from the semi-structured and unstructured documents, AI can also extract data from handwritten documents. The deep intricacies and capabilities of machine learning and deep learning enable the OCR algorithms to train themselves to understand the context, perform better classification and improve accuracy.
Validation – With an extensive database and an integrated system, AI can validate the data it has extracted. AI-powered OCR can perform multi-way searches, which involve matching multiple fields to an item in the back-end system. This means, that even if an item doesn’t match with the data in the database, AI can still perform a multi-way search to find the same item.
AI-based OCR tools can analyze and interpret the content of the documents they have extracted. The efficiency of an AI-based OCR system is significantly improved by the amount of data it can learn from.
Devfi, a digital transformation and AI technology company, can help in developing AI-powered OCR systems without any major hurdles. Before deploying an OCR solution, we consider a few aspects like the input material (ensuring that the input data is free of damage and is suitable for the OCR algorithms), training dataset (to train or retrain the model with accurate and annotated data), performance monitoring (after deployment and retraining the model with the real-time data to maintain the accuracy levels).
For more information on how we can conduct a thorough evaluation of your business requirements to determine the best OCR option, feel free to contact us.
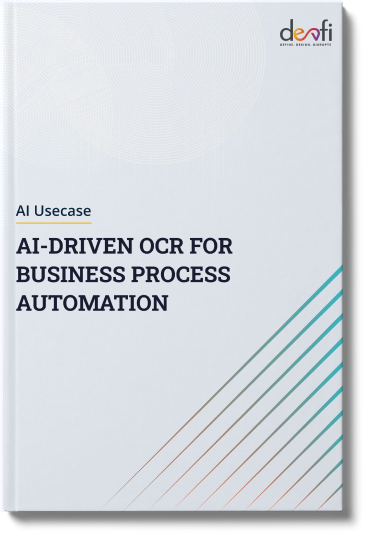